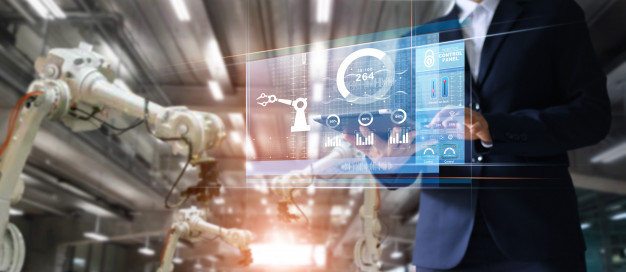
The fourth industrial revolution, or Industry 4.0 as it has become known, is already in full swing. Like the steam engine that ushered in the Industrial Revolution in the mid-eighteenth century, Industry 4.0 is continuously redefining the way we manufacture goods by automating traditionally manual processes by leveraging computerization and other modern technologies. In much the same way the steam engine has become synonymous with the start of one of the most transformative times in human history, “smart factories” – modern factories in which physical production methods and operations are combined with digital technology, smart computing, and machine learning – are defining this latest industrial revolution. As Industry 4.0 progresses and smart factories steadily become the norm, artificial intelligence – or more specifically, machine learning – will play an increasingly important role when it comes to careers in robotics. On that note, let’s take a closer look at machine learning and how it can contribute to smart manufacturing.
What is Machine Learning?
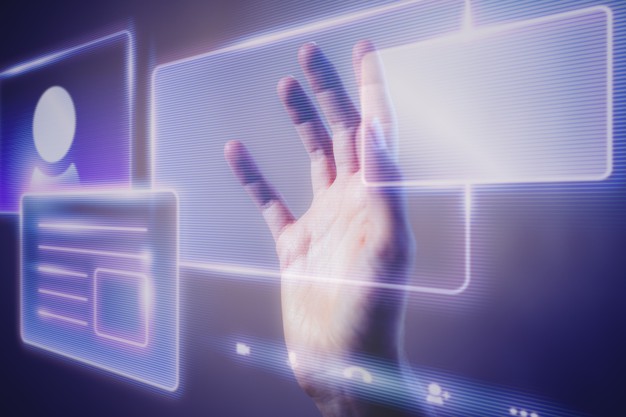
The term artificial intelligence, or AI for short, is probably something that most of us are familiar with or at least aware of. Machine learning is a facet of technology that falls within the AI umbrella, but what does it mean? Don’t all computerized machines “learn” how to perform a task once the programmer finishes writing, testing, and uploading the code? The truth is, machine learning is substantively different than simply writing new code for a given task. Machine learning allows systems to automatically learn and improve upon its processes from experience without explicitly being programmed to do so. If it sounds like a wild idea, it’s because it is. At its core, machine learning can be divided into three distinct approaches, which we’ll briefly summarize below.
- Supervised Learning. In this approach, the computer is presented with a series of sample inputs and associated outputs by a human operator. The idea here is that the computer will begin to learn how inputs and outputs are mapped to one another (e.g. input A results in output A, input B results in output B, etc.). In this approach, historical input and output pairs are presented which allows the computer to learn and “understand” the relationship between the two. Once the connection is made, additional inputs are fed into the computer which in turn can “predict” the appropriate output.
- Unsupervised Learning. In this approach, only inputs are presented to the computer; sample input/output pairs are not provided, meaning the computer is left to discover an associated output. Though this may sound like an inefficient way for a machine to learn, unsupervised learning can yield some interesting hidden patterns within the data. Admittedly, unsupervised learning approach takes more time than the supervised learning approach because the problem is less defined. Think about how quickly you could become a master martial artist under the tutelage of a sensei compared to how long it would take if you were to teach yourself. You might have an idea on how to complete a form, but your sensei has the knowledge and experience to correct any errors you make. Unsupervised learning is often used to cluster data into groups that feature differing characteristics.
- Reinforcement Learning. Less common than the other learning approaches and arguably much more complex, reinforcement learning uses what can best be described as a “reward system” to teach the computer system to perform an action and achieve a desired outcome. As it navigates its problem space, the program is provided feedback that’s analogous to rewards, which it tries to maximize. If you haven’t already, think of this approach as one you might take when training a dog to perform a particular task. Good behaviour that is rewarded with praise, treat, or a toy becomes common; punishing undesirable behaviour results in the behaviour becoming less common.
Machine Learning and Smart Manufacturing
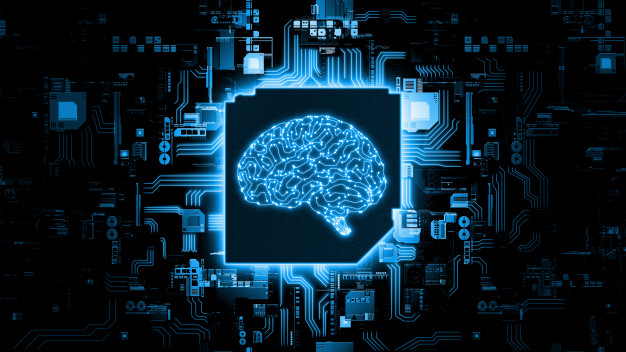
In the world of smart manufacturing, machine learning will be fundamental for optimizing production processes, reducing lead times and reducing energy consumption. There are several ways in which machine learning techniques are being applied in modern smart factories.
- Quality Control. By analyzing vast amounts of data, machine learning plays a critical role in enhancing Overall Equipment Effectiveness (OEE) – the gold standard in manufacturing. To put it simply, OEE measures the manufacturing time that is productive; to have an OEE score of 100% would mean that only good products are produced, in the least amount of time, with no down time. With machine learning, the system can quickly identify weaknesses in various pieces of equipment and help to minimize those weaknesses.
- Perfecting the Supply Chain. Being able to successfully manage inventory and supply chains is an endeavor that can have a major impact on a manufacturer’s bottom line. If either is inefficiently managed, a company could end up with too much inventory or not enough raw materials to maintain production. Machine learning can help maximize asset management and inventory management systems by predicting the exact moment additional resources will be needed.
- Optimized Semiconductor Manufacturing. Machine learning uses root-cause analysis to reduce testing costs through streamlining manufacturing workflows. Manufacturing equipment that runs on ML technology is expected to be 10% cheaper in annual maintenance expenses.
Challenges for the Future
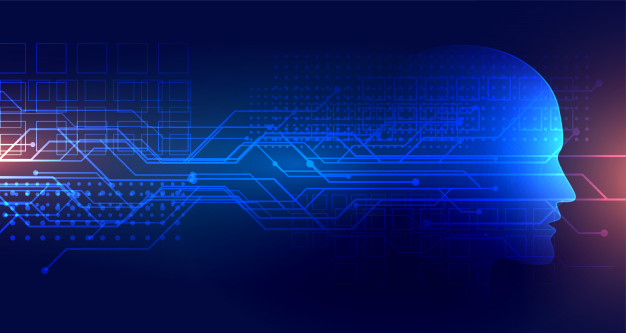
The technology that drives machine learning may be expanding at a rate commensurate with other transformative technologies, but it’s important to remember that it is still a relatively new subset of the much broader artificial intelligence. Like any other new technologies, there are bound to be some hurdles that will need to be overcome.
- Integration. Replacing large industrial equipment is expensive and that means that manufacturers tend to hold on to them for as long as possible. For smaller manufacturers in particular, it can be a hard sell to replace machinery that has, and continues to, function admirably. In these cases, the cost of integrating machine learning into a system can be a barrier to entry for many small and mid cap manufacturers.
- Security. The interconnection of manufacturing components poses a great risk to the security of the entire processing plant. In cases where machines are working alongside humans, it could result in exposing them to danger if the machines are compromised.
- Skills in Companies. Since machine learning is a new technology, many manufacturers are faced with a unique human resource dilemma: recruit new staff with the right knowledge or training the existing staff in the smart manufacturing environment. Since new industrial automation equipment can displace workers that have traditionally completed manual tasks, it might be beneficial to leverage the knowledge of existing workers and train them for new, more meaningful and rewarding roles within the company.
Lean, Mean, Learning Machines
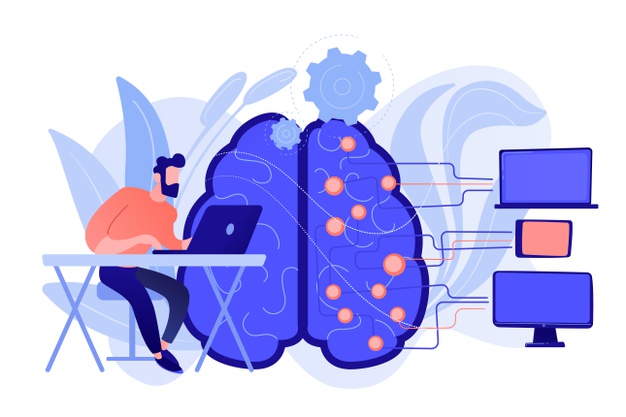
Though in its relative infancy, machine learning presents an exciting new opportunity for Industry 4.0. As it continues to proliferate throughout the manufacturing sector, not only will processes be made more efficient, but new, exciting employment opportunities will become available. If you’re interested in robotics technician training, be sure to check out what GBCTechTraining has to offer.